How Historical Data Improves Casting Automation
Explore how historical data enhances casting automation, improving efficiency, role matching, and overall success for actors.
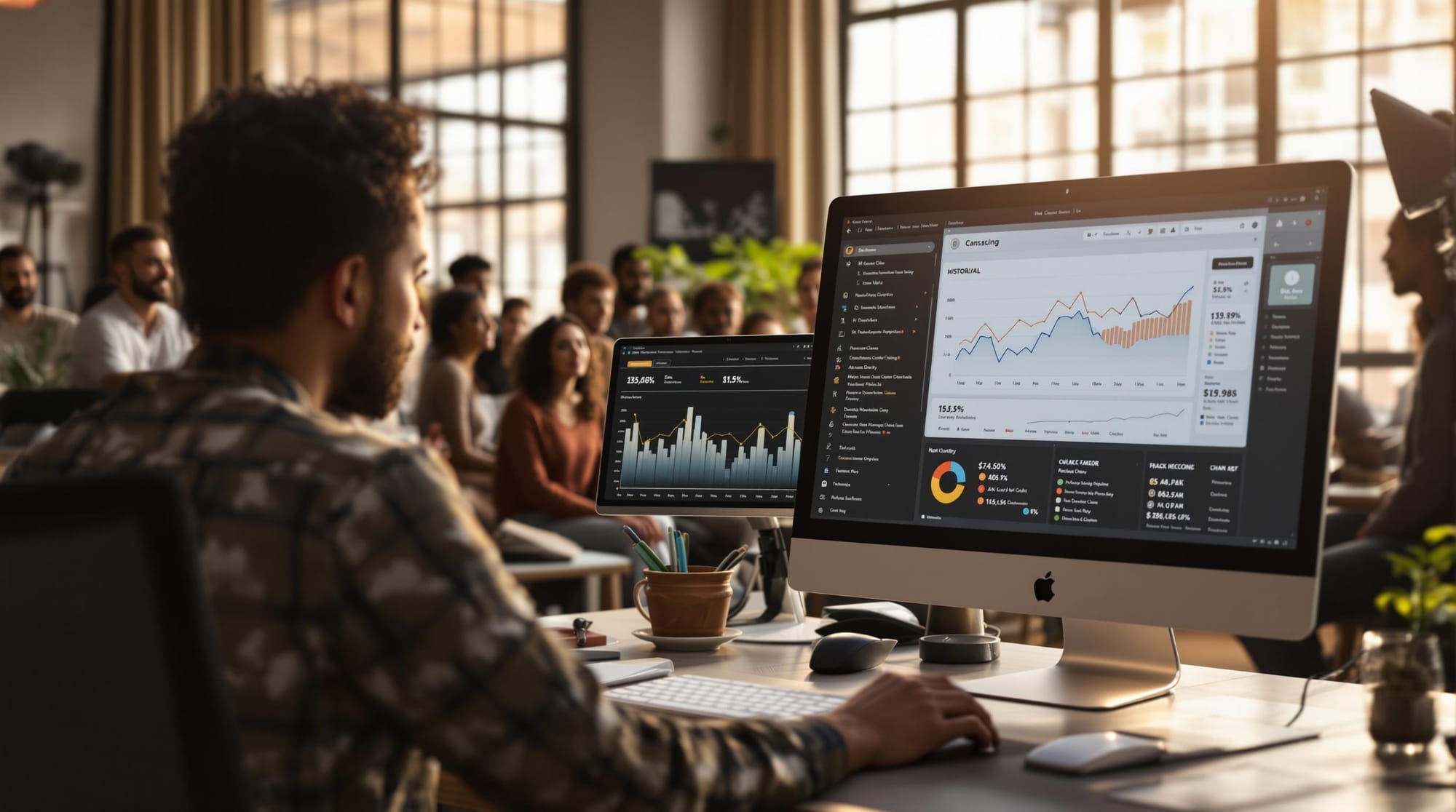
Using historical data has transformed casting automation, making it faster and more accurate. Here's how:
- Saves Time: Automation reduces manual work, saving actors over 5 hours per week on average.
- Better Role Matching: Platforms analyze past applications, preferences, and outcomes to suggest roles that fit actors' profiles.
- Improved Results: Higher callback rates and more bookings for actors using automated systems like CastmeNow.
- Convenience: Features like auto-apply and unified profiles streamline the process, letting actors focus on their craft.
For example, actors like Sophie M. and Daniel K. report getting more callbacks and finding roles easily with tools like CastmeNow. By leveraging historical data, casting platforms make the process faster, smarter, and more effective.
Your Entire Actor Database in One Place
Understanding Historical Data in Casting
Historical data - such as actors' past experiences, preferences, and outcomes - forms the backbone of matching systems that pair talent with the right roles.
Types of Historical Data
Casting platforms use various types of historical data to refine their processes and improve results. Here's a breakdown:
Data Type | Description | Impact on Automation |
---|---|---|
Actor Preferences | Role types, locations, and pay rates | Filters out irrelevant opportunities |
Application Patterns | Submission timing, frequency, and response rates | Optimizes when and how submissions occur |
Success Metrics | Callback rates and booking statistics | Increases matching precision |
Role Requirements | Character descriptions, physical attributes, and skills | Ensures better alignment with roles |
Together, these data points create a detailed profile that powers automated decision-making.
"I used to spend so much time scrolling through casting websites looking for Voiceover roles. With CastmeNow, I simply set my preferences, and it applies for me. I've never felt more in control of my time!"
Impact on Casting Results
The use of historical data plays a big role in improving casting outcomes. Here’s how:
- Learning Preferences: Automated systems analyze past applications to identify roles that align with an actor's goals. This minimizes time wasted on unsuitable opportunities and increases the chances of finding a good match.
- Spotting Patterns: By examining successful castings, platforms uncover trends that fine-tune their matching process.
- Maximizing Time Efficiency: Historical data helps identify the best times to submit applications, boosting visibility with casting directors.
For instance, CastmeNow uses historical data for real-time profile updates, incorporating factors like union filters and size cards to enhance role matching. These insights set the stage for further advancements in casting tools, which will be explored next.
Data-Driven Improvements in Casting Tools
Smarter Role Recommendations
Modern casting platforms now use historical data to offer tailored role suggestions. By studying past preferences and application trends, these tools create advanced matching systems. For example, CastmeNow's "Unified Profile Hub" builds detailed profiles by linking multiple casting accounts automatically.
Here's how the system works to ensure better role matching:
Data Component | Purpose | Benefit to Actors |
---|---|---|
Profile Details | Syncs info from connected accounts | Ensures consistent representation everywhere |
Past Applications | Reviews submission history | Highlights patterns in successful matches |
User Preferences | Tracks role types and requirements | Fine-tunes future recommendations |
This data-driven approach not only boosts accuracy in role matching but also speeds up application processing.
Faster and More Efficient Applications
Automation powered by data has made applying for roles quicker and more targeted. Instead of manually combing through endless listings, actors can now rely on smart systems to submit applications based on their specific preferences.
Platforms like CastmeNow offer plans to streamline this process:
- Basic Plan ($3/month): Handles up to 150 submissions per month.
- Unlimited Plan ($8/month): Offers unlimited submissions and broader platform integration.
These tools save time, allowing actors to focus on their craft while the technology handles the heavy lifting.
Predicting Future Opportunities
Historical data isn't just for matching - it also helps forecast future casting trends. These predictive insights provide a strategic edge by:
- Analyzing seasonal patterns in casting
- Tracking shifts in role requirements across the industry
- Studying success rates for different role types
- Identifying the best times to submit applications
Common Data Issues and Fixes
Keeping data accurate and managing processing challenges are critical for ensuring automated systems work efficiently and meet user needs. While historical data is the backbone of modern casting automation, certain challenges need careful attention to maintain system performance.
Keeping Data Accurate
The quality of data directly affects how well automated casting systems match actors to roles. Platforms use various methods to ensure data stays reliable and useful:
Quality Issue | Solution | Impact |
---|---|---|
Profile Inconsistencies | Unified Profile Hub sync | Ensures consistent details across platforms |
Outdated Information | Auto profile refresh | Keeps profiles up to date |
Incomplete Data | Smart completion prompts | Improves match precision |
These measures have proven effective, with actors reporting better matches and saving time thanks to these quality controls.
Handling Large Data Volumes
Modern casting platforms process massive amounts of data while maintaining speed and reliability. They prioritize relevant matches through intelligent data handling, which includes:
- Managing thousands of role listings every day
- Integrating multiple platforms at the same time
- Running advanced matching algorithms in real-time
In addition to managing large datasets, these platforms must strike a balance between automated processes and user input.
Blending Automation with Personal Input
Finding the right mix of automation and personal control is essential for successful casting submissions. Platforms address this by:
- Using smart filters that combine historical data with user preferences
- Making real-time adjustments based on patterns of success
- Allowing users to customize and control automated processes
Sophie M., a film and television actor, highlights the benefits:
"As a working actor on set 10+ hours a day, I needed something that could keep me in the game without taking up my entire day. CastmeNow's automation lets me stay in front of casting directors without the hassle."
These approaches show how platforms can merge data-driven automation with personal preferences, making the casting process both efficient and user-friendly.
CastmeNow: Real-World Application
CastmeNow shows how historical data can transform the casting process by enabling accurate role matching and fast application handling. Its data-focused tools bring automation to the acting world.
Main Features of CastmeNow
The platform combines historical data analysis with automation through three key features:
Feature | Function | Data Usage |
---|---|---|
Smart Auto-Apply | Automatically submits applications for roles | Uses user preferences, union filters, and past application trends |
Unified Profile Hub | Synchronizes profiles across platforms | Merges casting data from multiple sources for better matching |
Discover & Apply | Scores roles based on relevance | Leverages historical casting data to create fit scores |
These features allow the platform to continuously improve its matching process using historical data.
Data Analysis Methods
CastmeNow employs several advanced techniques to process casting data effectively:
- Profile Integration: Automatically imports and analyzes data from connected casting accounts to create detailed actor profiles.
- Fit Score Generation: Uses historical data to calculate fit scores, ranking roles based on their relevance to the user.
- Preference Learning: Adapts to user behavior over time, fine-tuning its matching algorithm by analyzing application habits.
These methods lead to noticeable improvements in the casting experience for actors.
User Results
Actors have seen real, practical benefits from this approach. Emma L., a voiceover artist from New York, shares:
"I used to spend so much time scrolling through casting websites looking for Voiceover roles. With CastmeNow, I simply set my preferences, and it applies for me. I've never felt more in control of my time!"
Conclusion
Casting automation has taken a huge leap forward by using historical data to replace manual processes with efficient algorithms. These systems analyze user preferences and past application trends, saving actors over 5 hours per week on average.
With these data-driven advancements already proving their worth, the future of casting automation looks promising. As algorithms improve, these systems will become even better at matching actors with roles, simplifying the process at every step.